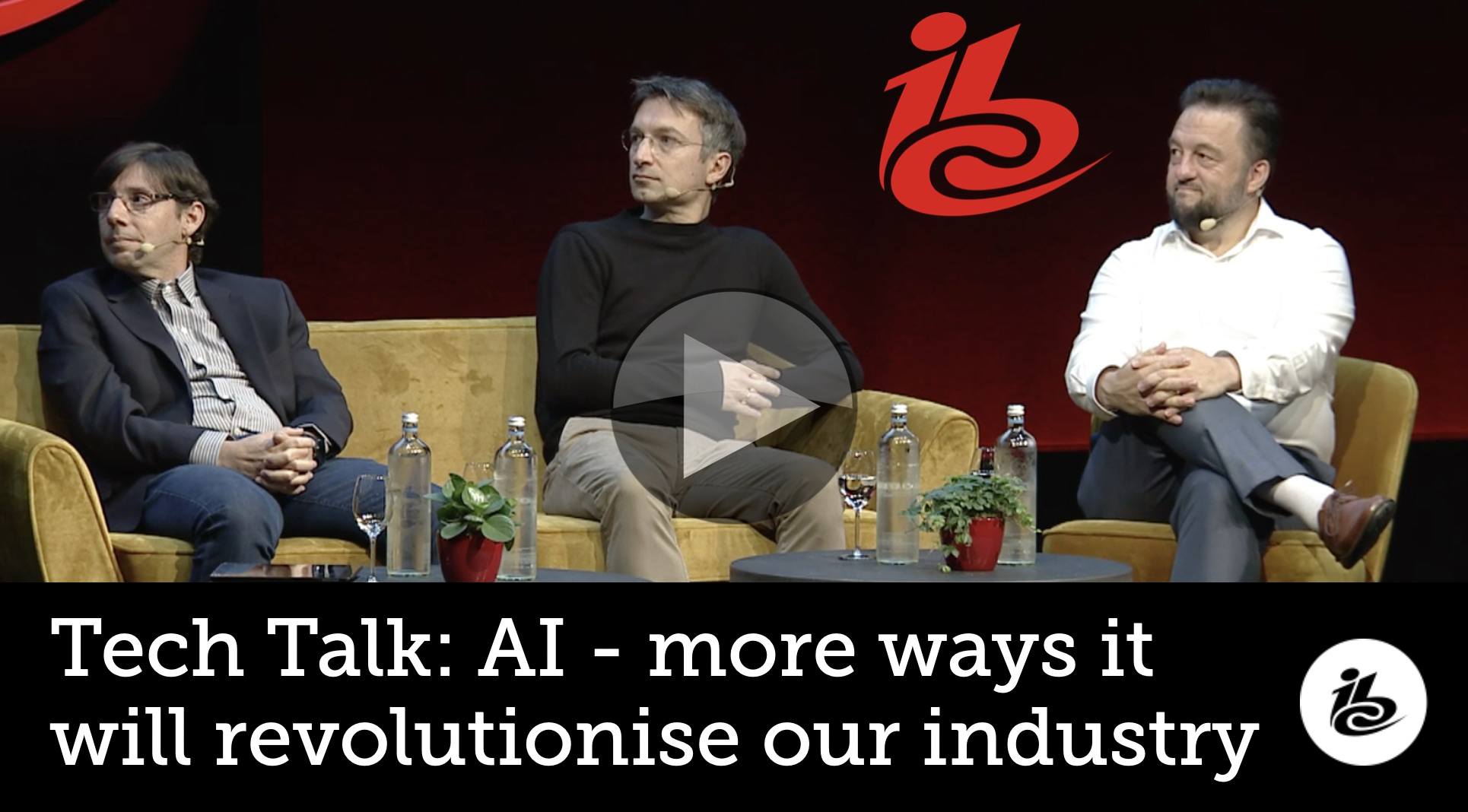
Artificial Intelligence and Machine Learning (ML) dominate many discussions and for good reason, they usually reduce time and reduce costs. In the broadcast industry their are some obvious areas where it will, an already does, help. But what’s the time table? Where are we now? And what are we trying to achieve with the technology?
Edmundo Hoyle from TV Globo explains how they have managed to transform the thumbnail selection for their OTT service from a manual process taking an editor 15 minutes per video to an automated process using machine learning. A good thumbnail is relevant, it is a clear picture and has no nudity or weapons in it. Edmundo explains that they tackled this in a three-step process. The first step uses NLP analysis of the episode summary to understand what’s relevant and to match that with the subtitles (closed captions). Doing this identifies times int he video which should be examined more closely for thumbnails.
The durations identified by the process are then analysed for blur-free frames (amongst other metrics to detect clear videography) which gives them candidate pictures which may contain problematic imagery. the AWS service Rekognition which returns information regarding whether faces, guns or nudity are present in the frame. Edmundo finishes by showing the results which are, in general very positive. Final choice of thumbnails is still moderated by editors, but the process is much more streamlined because they are much less likely to have to find an image manually since the process selects 4 options. Edmundo finishes by explaining some of the chief causes of rejecting an image which are all relatively easy to improve upon and tend to be related to a person looking down or away from the camera.
We’ve seen before on The Broadcast Knowledge the idea of super-resolution which involves up-scaling images/video using machine learning. The result is better than using standard linear filters like lanczos. This is has been covered in a talk from Mux’s Nick Chadwick about LCEVC. Yiannis Andreopoulos from iSize talks next about the machine learning they use to improve video which uses some of these same principles to pre-treat, or as they call it ‘pre-code’ video before it’s encoded using a standard MPEG encoder (whether that be AVC, HEVC or the upcoming VVC). Yiannis explains how they are able to understand the best resolutions to encode at and scale the image intelligently appropriately. This delivers significant gains across all the metrics leading to bandwidth reduction. Furthermore he outlines a system which feeds back to maintain both the structure of the video which avoids it becoming too blurry which can be a consequence of being to subservient to the drive to reduce bitrate and thus simplifying the picture. It can also, though, protect itself from going too far down the sharpness path and only chasing metrics gains. He concludes by outlining future plans.
Grant Franklin Totten then steps up to explain how Al Jazeera have used AI/machine learning to help automate editorial compliance processes. He introduces the idea of ‘Contextual Video Metadata’ which ads a level of context to what would otherwise be stand-alone metadata. To understand this, we need to learn more about what Al Jazeera is trying to achieve.
As a news organisation, Al Jazeera has many aspects of reporting to balance. They are particularly on the look out for bias, good fact-checking & fake news. In order to support this, they are using AI and machine learning. They have both textual and video-based methods of detecting fake news. As an example of their search for bias, they have implemented voice detection and analysed MP’s speech time in Ireland. Irish law requires equal speaking time, yet Al Jazeera can easily show that some MPs get far more time than others. Another challenge is detecting incorrect on-screen text with the example given of naming Trump as Obama by accident on a lower-third graphic. Using OCR, NLP and Face recognition, they can flag issues with the hope the they can be corrected before Tx. In terms of understanding, for example, who is president, Al Jazeera is in the process of refining the Knowledge graph to capture the information they need to check against.
AI and machine learning (ML) aren’t going anywhere. This talk shines a light on two areas where it’s particularly helpful in broadcast. You can count on hearing significant improvements in AI and ML’s effectiveness in the next few years and it’s march into other parts of the workflow.
Watch now!
Speakers
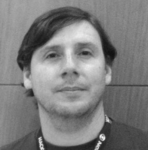 |
Edmundo Hoyle
TV System Researcher
TV Globo
|
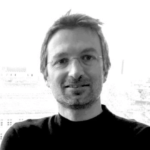 |
Yiannis Andreopoulos
Technical Director,
iSize Technologies
|
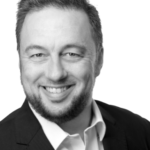 |
Grant Franklin Totten
Head of Media & Emerging Platforms,
Al Jazeera Media Networks
|
Edmundo Hoyle (GLOBO), Yiannis Andreopoulos (iSize Technologies) and Grant Totten (Al Jazeera Media Network).